Project Team
- Taejoon Kim (Arizona State University)
- David Love (Purdue University)
Project Vision
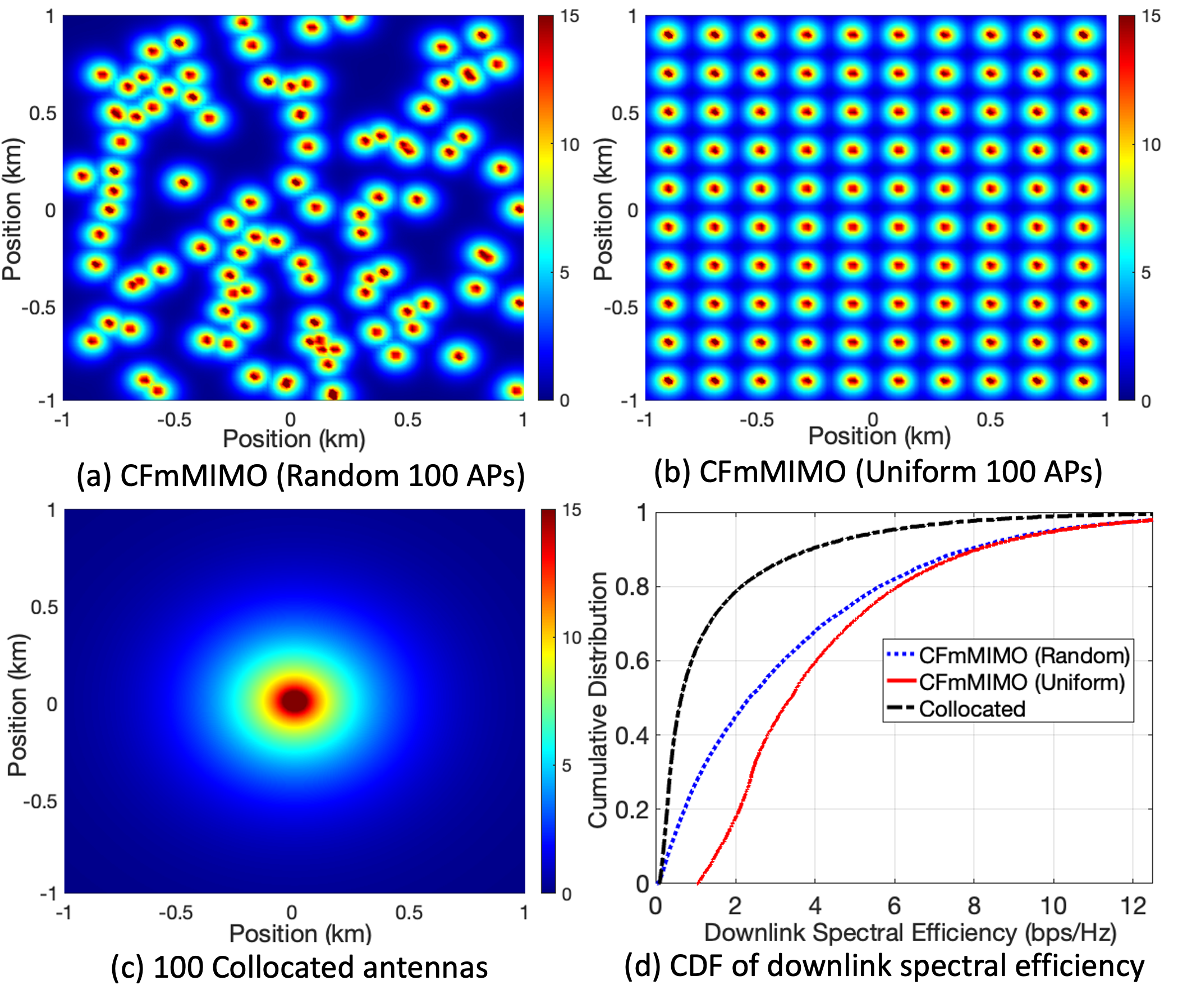
Over the last few years, discussions oriented toward defining sixth generation (6G) requirements and possible technologies have started to circulate within the wireless community. One of the key ideas will likely be to take steps to remove the conventional cell boundaries and facilitate enhanced joint uplink and downlink processing using many dispersed access points (APs). These ideas fall within the academic definition of cell-free massive multiple-input multiple-output (CFmMIMO). It alleviates the existing cell-edge and handover problems and improves energy efficiency. The primary limiting factor is achieving cell-free operation in a practically feasible way, with computational complexity and fronthaul requirements that are scalable to large networks with many users. This poses many important research questions that must be explored systematically and in-depth. This project firstly develops scalable artificial intelligence (AI)-based solutions. Together with the appropriate (cost-efficient) AP deployment planning tools (e.g., where to put the APs), these developments constitute a significant step toward enabling the low-latency and uniformly reliable wireless services at a lower cost. Given the international nature of the project, the project contributes to the development of a diverse workforce in AI and 6G wireless networks through the formation of international research teams integrating undergraduate and graduate students.
The goal is to provide the algorithmic foundations of cell-free massive multiple-input multiple-output (CFmMIMO) networks so that CFmMIMO networks scale with the number of APs and the number of users, without taking the computational complexity and coordination overhead for granted. This fundamental research occurs while imposing practical spectrum, geographic, and security constraints that hamper real-world network spectral efficiencies and are usually ignored by the academic community. We aim to address several significant novel aspects on the way towards scalable solutions beyond 5G RANs. To achieve this, the following major goals are considered:
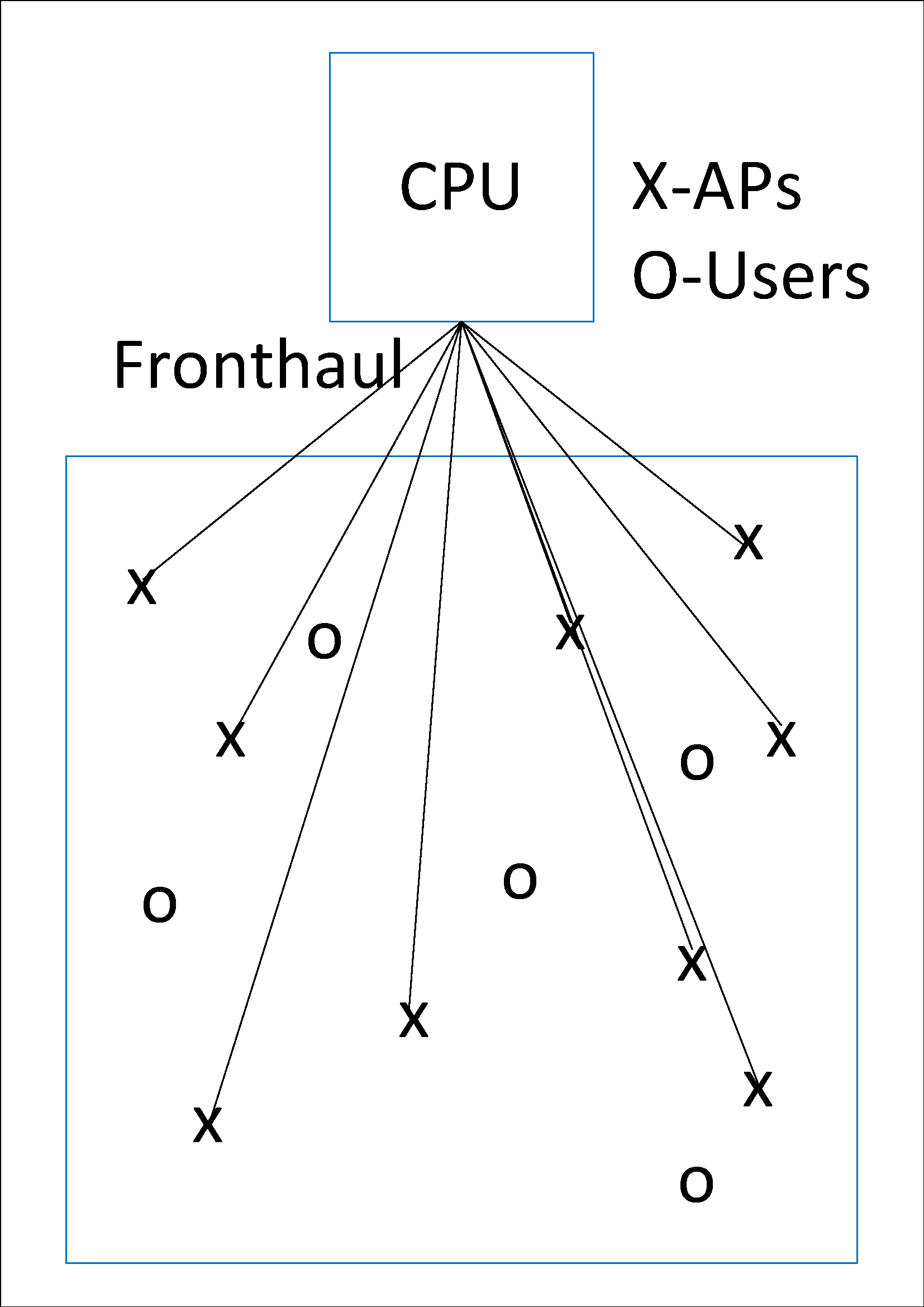
Goal 1–Scalable AI-Based Solutions for CFmMIMO: This thrust focuses on developing AI-based data-driven scalable solutions for CFmMIMO. Particularly, we will develop AI-based power control and pilot assignment solutions enabling the implementation of large-scale CFmMIMO. We will investigate truly scalable data-driven approaches and AI architectures applicable to large networks with many users. The security aspects, especially in the context of AI algorithms and architectures, will also be investigated.
Goal 2–Scalable Deployment Solutions for CFmMIMO: This thrust focuses on establishing network planning and waveform constraints to address scalable deployment solutions for CFmMIMO. We will investigate infrastructure-aware minimum-cost AP deployment methodologies by taking into account the QoS requirements and available transport infrastructure. We will then investigate how CFmMIMO can enable itself to be deployed in bands that are close to legacy bands and potentially address many of today's most challenging spectrum policy issues.
Evaluation Thrust: We will utilize existing simulation and experimental capabilities to analyze these systems and provide a continuous feedback cycle between theory and experimentation.
In addition to the Intellectual Merits, this project that aims to provide algorithmic foundations for CFmMIMO networks can be a centerpiece of technology innovation, as it can provide foundations for economic growth. It is also instrumental in developing 5G systems and beyond. The PIs will actively collaborate with industrial collaborators in technology transfer, joint patent filings, publications, and commercialization of the proposed research. The PIs will build on their track records of engaging students from underrepresented and minority groups in research. The results will be integrated with undergraduate- and graduate-level course offerings. Dissemination of the research results through the communications, computing, and agricultural areas will spur further development at the intersection of these communities.
Intellectural Merit
To address Goal 1, we divided our research efforts into the following tasks in Year 1 of the project:- In the first research task, we began by designing the CFmMIMO system based on the ORAN architecture. We specifically focused on channel estimation and pilot assignment (PA) models considering practical aspects (e.g., fronthaul overhead and operational complexity by each functional unit), which can be adapted to the O-RAN CFmMIMO systems. We then designed a Markov game model for our multi-agent deep reinforcement learning (MA-DRL) which leads to an efficient solution for our decentralized PA problem. In particular, we formulate our reward based on observations directly measurable at the Open Radio Units (O-RUs). Thus, our scheme does not require prior knowledge of channel statistics. By adopting the MA-DRL framework, we proposed a novel learning-based PA scheme aiming to minimize the total mean squared error (MSE) across the users. We designed two effective ways to improve the PA performance: (i) inter-DU message passing for observation sharing and (ii) a low-complexity codebook search (CS) algorithm. We numerically evaluated the performance of our PA scheme against the state-of-the-art in both channel estimation performance and computational complexity.
- In the first research task, we embarked on the introduction of a model for the elementary events of link outage, which captures the randomness of physical blockage, UE access-limited blockage, and SINR outage. The developed model also accounts for directional beam patterns at each base station as well as random locations of obstacles and UEs. Using these models, we formulated the UE-outage-guaranteed minimum-cost RU deployment problem as a large-scale integer non-linear programming (INP). We conducted a bound analysis and showed that the UE outage constraint can be approximated as a set of analyzable constraints. By doing so, we tractably handled the large-scale INP. We decomposed the formulated INP into two separable subproblems: (i) RU coverage optimization problem and (ii) minimum subset RU selection problem. Finally, we evaluated the efficacy of our proposed designs through numerical simulations.
- In the second research task, we first formulated a privacy-preserving (DP) learning framework as a differential-privacy-preserving wireless federated learning (FL) problem in CFmMIMO networks. Taking advantage of the existing wireless channel noises, we jointly determined the DP noise variance and users’ transmit power values to address privacy and learning utility tradeoffs. Next, we optimized the number of FL iterations by minimizing the upper bound on the learning error. We conducted simulations to demonstrate the efficacy of our approach in terms of DP guarantee and learning utility.
- In the third research task, we formulated a technique to utilize new bands without interfering with legacy wireless users. The sub-6 GHz band is heavily populated, and introduction of new bands has the potential to interfere with legacy wireless users since the receivers were designed based on spectral usage in the past. The legacy users are typically located in a geographically constrained region, we use this information at the transmitter to limit the interference in the region. We achieve that by incorporating quadratic constraints called region constraints into the massive MIMO precoder optimization problem. We performed simulations to illustrate the efficacy of our approach.
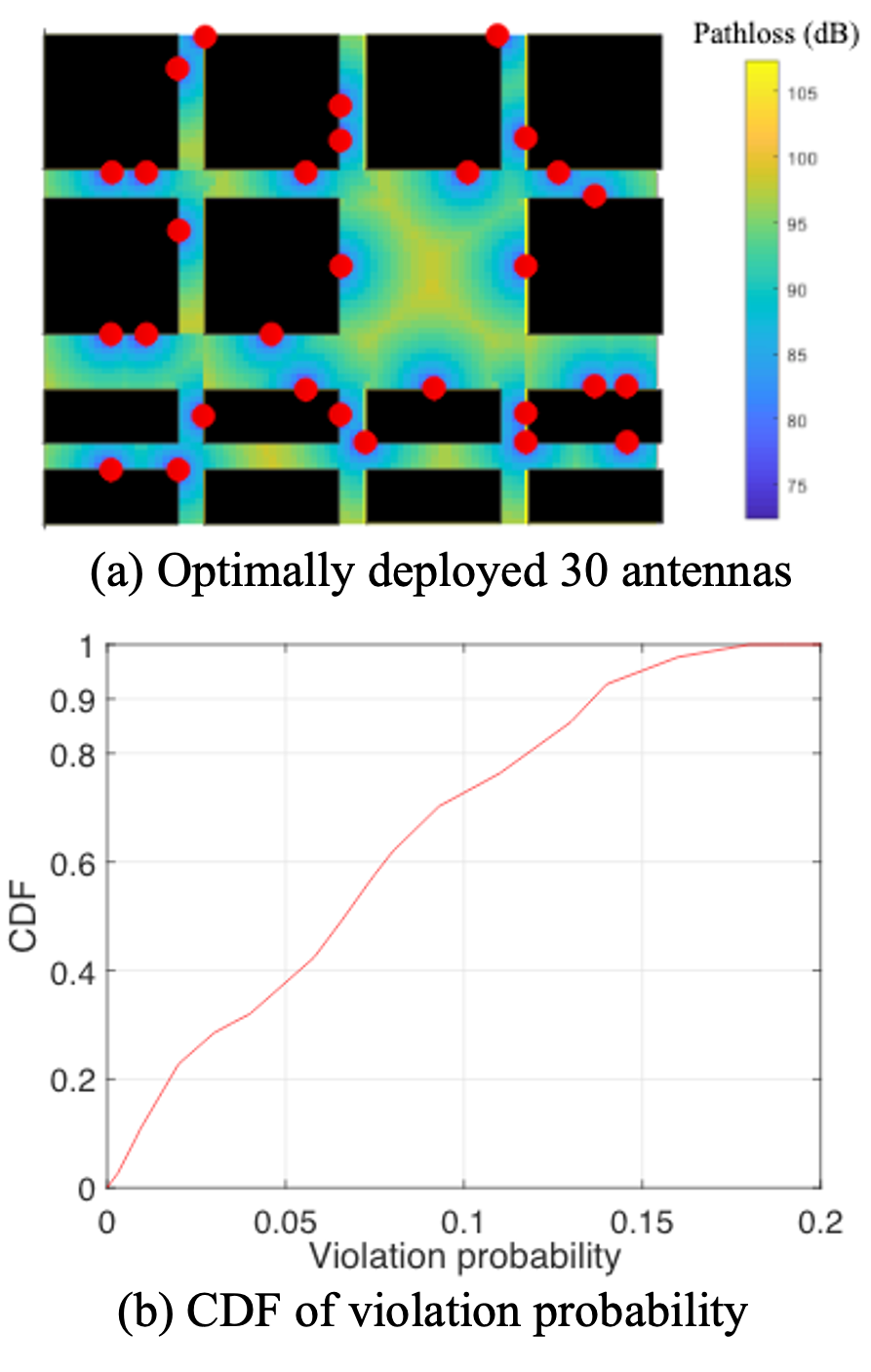
Broader Impacts Activities in Year 1 of The Project
This project has involved three graduate students across KU, Purdue, and Aalto University. Given the cross-institutionary nature of the project and integration of the developed methods, significant effort will target collaboration. The team has already implemented a successful working model in place via cross-institutional graduate student mentoring. The PIs Kim, Love, and Vorobyov have been holding monthly group meetings to track progress and co-mentor a cluster of Ph.D. students from each institution, which will contribute to future workforce development by creating cross-institutional research experience in signal processing, wireless communications, and security on 6G CFmMIMO networks.
Publications
- M. S. Oh, A. B. Das, S. Hosseinalipour, T. Kim, D. J. Love, and C. G. Brinton, “A Decentralized Pilot Assignment Methodology for Scalable O-RAN Cell-Free Massive MIMO,” IEEE Journal on Selected Areas in Communications, December 2023.
- M. Dong, M. Cho, K. Lee, S. Yoon, and T. Kim, “Cost-Optimal Deployment of Millimeter-Wave Base Stations Under Outage Requirement”, IEEE Transactions on Wireless Communications, December 2022.
- D. Q. Nguyen and T. Kim, “Time-Varying Noise Variance Perturbation and Power Control for Privacy-Preserving Wireless Federated Learning,” IEEE the 57th Asilomar Conference on Signals, Systems, and Computers, November 2023.
- D. Q. Nguyen and T. Kim, “On the Stability of Approximate Message Passing with Independent Measurement Ensembles,” IEEE International Symposium on Information Theory (ISIT), June 2023.
- J. Kim, T. Kim, D. J. Love, and C. G. Brinton, "Robust Non-Linear Feedback Coding via Power-Constrained Deep Learning", International Conference on Machine Learning (ICML), 2023.
- W. Zhang and T. Kim, “Successful Recovery Performance Guarantees of Noisy SOMP,” IEEE Transactions on Vehicular Technology, December 2023.
- M. S. Oh, A. Bijoy Das, T. Kim, D. J. Love and C. G. Brinton, “Minimum Description Feature Selection for Complexity Reduction in Machine Learning-Based Wireless Positioning,” IEEE Journal on Selected Areas in Communications, vol. 42, no. 9, pp. 2585-2600, Sept. 2024.
- D. Q. Nguyen, A. Ashikhmin, H. Yang and T. Kim, “No Analog Combiner TTD-Based Hybrid Precoding for Multi-User Sub-THz Communications,” ICC 2024 - IEEE International Conference on Communications, Denver, CO, USA, 2024, pp. 1813-1818.
- M. S. Oh, A. B. Das, T. Kim, D. J. Love and C. G. Brinton, “Complexity Reduction in Machine Learning-Based Wireless Positioning: Minimum Description Features,” ICC 2024 - IEEE International Conference on Communications, Denver, CO, USA, 2024, pp. 2264-2269.
- O. M. Kandelusy and T. Kim, "Buffer-Aided Distributed Compressed Transmission and Fusion Under Energy and Reliability Constraints," IEEE Transactions on Green Communications and Networking, Nov. 2024.
- D. Q. Nguyen and T. Kim, “Joint Delay-Phase Precoding Under True-Time Delay Constraints in Wideband Sub-THz Hybrid Massive MIMO Systems,” IEEE Transactions on Communications, vol. 72, no. 10, pp. 6633-6646, Oct. 2024.
- J. Kim, T. Kim, A. B. Das, S. Hosseinalipour, D. J. Love, and C. G. Brinton, “Coding for Gaussian Two-Way Channels: Linear and Learning-Based Approaches,” submitted to IEEE Transactions on Information Theory, 2024.
- S. Mathad, T. Kim, and D. J. Love, “Protecting Legacy Wireless Systems Against Interference using Massive MIMO,” the 58th Asilomar Conference on Signals, Systems, and Computers, October 2024.
- H. Kim, H. Nam, and D. J. Love, "Robust Over-the-Air Federated Learning," 2024 58th Annual Conference on Information Sciences and Systems (CISS), Princeton, NJ, USA, 2024, pp. 1-6.
- A. P. Mohamed, B. Lee, Y. Zhang, M. Hollingsworth, C. R. Anderson, J. V. Krogmeier, and D. J. Love, “Simulation-enhanced data augmentation for machine learning pathloss prediction,” ICC 2024-IEEE International Conference on Communications. IEEE, 2024.
- B. Lee, A. B. Das, D. J. Love, C. G. Brinton, and J. V. Krogmeier, "Constant Modulus Waveform Design with Block-Level Interference Exploitation for DFRC Systems," ICC 2024 - IEEE International Conference on Communications, Denver, CO, USA, 2024, pp. 324-329.
- V. Rana, R. A. Chou and T. Kim, "Short Blocklength Secret Coding via Helper-Assisted Learning over the Wiretap Channel," ICC 2024 - IEEE International Conference on Communications, Denver, CO, USA, 2024, pp. 1485-1490.
- B. Lee, H. Kim, D. J. Love, and J. V. Krogmeier, “Spatial-division ISAC: A practical waveform design strategy via null-space superimposition,” Submitted to IEEE Transactions on Wireless Communications.
- A. N. Arun, B. Lee, F. A. Castiblanco, D. R. Buckmaster, C. C. Wang, D. J. Love, J. V. Krogmeier, M. M. Butt, and A. Ghosh, “Ambient IoT: Communications enabling precision agriculture,” Submitted to IEEE Communications Magazine.
- B. Lee, A. B. Das, D. Love, C. Brinton, and J. Krogmeier, “Constant modulus waveform design with interference exploitation for DRFC systems: A block-level optimization approach,” Submitted to IEEE Transactions on Signal Processing.
- S. Mathad, T. Kim, and D. J. Love, “Protecting legacy wireless systems against interference using massive MIMO and region constraints,” Submitted to IEEE Transactions on Wireless Communications.
Graduate Research Assistants & Collaborators
Graduate Research Assistants:
- Dang Qua Nguyen
- Myeung Suk Oh
- Byunghyun Lee
- Sameer Mathad
Collaborators:
- Sergiy Vorobyov (Aalto, Finland)
- James V. Krogmeier (Purdue)